After a summer break (while focusing on our 12 Clusters of Tech report) we are back with our Ethics in Tech Roundup! This time we're outlining some of the key insights, news articles & research pieces released in August and September 2020.

Amsterdam and Helsinki introduce open AI register
As places are becoming more digitised, Amsterdam and Helsinki have become the first two cities in the world to launch open AI registers aimed at improving public understanding of how their cities are using algorithms. Published information will include details on the data used by algorithms, their operating logic, and AI governance. Members of the public are also invited to provide feedback on the systems.
While this is a great initiative, it doesn’t look like it is compulsory for cities to release every app or algorithm onto the register - as reflected by the limited number of apps on the beta version. The cities are planning on expanding the register as the app evolves, but it would be interesting to see how representative the database will become. Read the press release on Cision (via TNW).
Biased algorithms used by the UK government are unfairly impacting people's lives
August and September featured two prominent stories of algorithms used by the UK government that have unfairly impacted people's lives by affecting allocation of grades and money.
Allocation of Grades
A common story now for many: In the wake of Covid-19, algorithms (paired with teacher assessments) were used to predetermine student grades. Sadly, there is no shortage of examples where students found their grades unfairly downgraded for reasons beyond their own control. The story provides a chilling example of how life-changing algorithms can be deployed without rigid testing of outcome accuracy and its potential for causing disproportionately biased outcomes. Furthermore, it reflects the dangers of using algorithms as means to determine human potential. Read more on this at Wired.
Allocation of Finance
International watchdog ‘Human Rights Watch’ has detected a flaw in the algorithm that is being used to calculate the allocation of UK benefits. The algorithm bases its calculations on the total wage received by applicants within a calendar month and doesn't take the frequency of payments into account. What this means is that if someone receives multiple pay checks in a month, as can be common in periods of irregular work, the algorithm can overestimate that person's earnings and shrink total benefit payments.
Instead, Human Rights Watch calls for a system that assesses shorter periods of income assessment or averaged earnings over longer periods of time. Read more on The Independent (thx TNW).
Microsoft watermarks images & deepfakes to improve content trustworthiness
Microsoft is developing a new system to combat fake imagery on the internet and restore (or build, depending on how skeptical you are) our trust in news sources. The system will enable image creators to ‘watermark’ online media through the use of digital hashes and certificates. These watermarks are then read by a browser extension, which will inform users whether the images they're seeing are authentic and whether any alterations have been made. The technology is to be tested by Project Origin: a collaboration between the BBC, CBC/Radio Canada, Microsoft and the New York Times. Microsoft is hoping the tool will become part of a wider verification standard. Read more at TechCrunch.
Court ruling: facial tech OK for greater good, but ethical use must be assessed
The South Wales Police (SWP) has lost a legal case on the way it was using facial recognition technology (FRT). The SWP had been using FRT to identify individuals listed on the police's Watchlist. To identify suspects, the FRT system would ‘scan’ all individuals in a public space and compare them with individuals listed on the SWP’s watchlist. If individuals were not featured on the list, they'd be immediately deleted from the system. However, this still means their information was captured and stored for a (very) brief period of time. While the court found no issue with deployment of FRT for purposes of the greater good, it did ask for a more rigorous and transparent assessment regarding the deployment of FRT, including information on:
- How to decide who is included on the watchlist
- Information on where the technology can be deployed
- Data protection impact assessment which assesses how potentially harmful outcomes can be prevented or mitigated
- Assessment of potential bias in the AI
Read more at TechCrunch.
Google starting ethics consultancy for AI companies
Google is planning to launch an AI ethics service before the end of the year to help organisations identify racial bias in algorithms and develop ethical guidelines. While Google has already been advising some companies on tech ethics, there are still challenges to the 'official' delivery of the service. One of them, as Wired has pointed out, is the balancing act of implementing thorough ethical AI processes and rapidly scaling a product/service to market. For example, a robust ethical review and design process could take 18 months to complete; a time during which competitors could move and gain foothold in the same market. Read more on Wired.
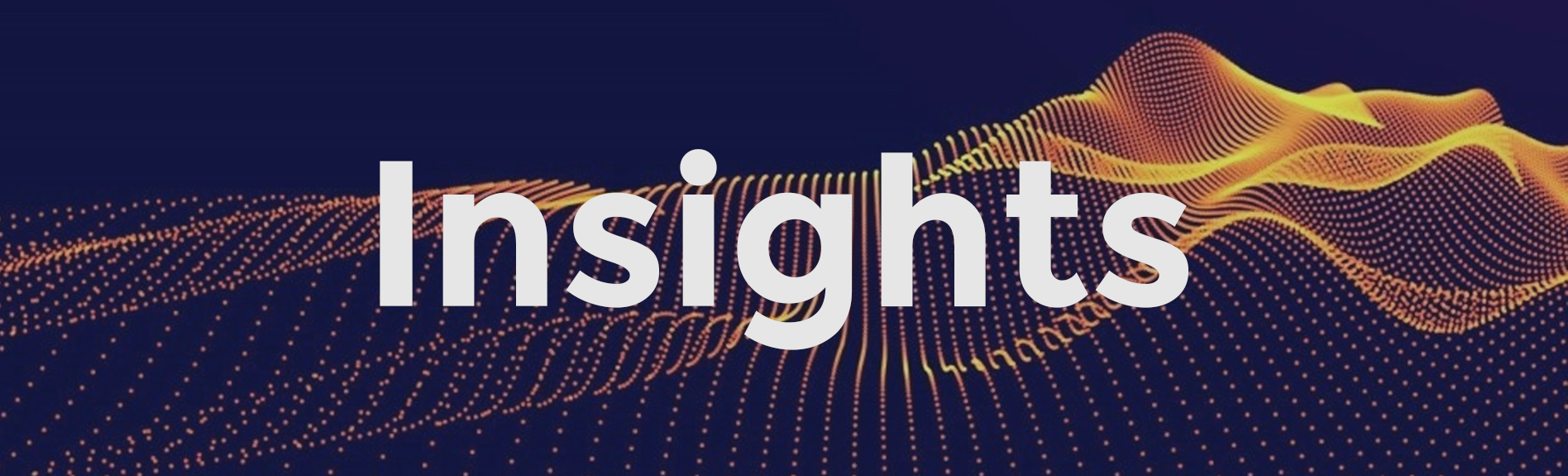
Ethical Dept Explained
Technology is neither good nor evil. Rather, the way that people use technology determines whether that technology could cause more good or harm. By considering how technologies could potentially be misused by bad actors, tech creators might be able to mitigate harmful outcomes. This is what is referred to in the concept of 'ethical debt'.
This article, on Wired, is a useful guide to understanding the importance and concept of ethical debt.
“As part of the design process, you should be imagining all of the misuses of your technology. And then you should design to make those misuses more difficult.
[..] Technologists often create “user personas” during the design process to imagine how different types of people might use that technology. If those personas don't include "user stalking their ex,” "user who wants to traumatize vulnerable people," and "user who thinks it's funny to show everyone their genitals,” then you're missing an important design step.”. Read more on Wired.
The algorithms that make big decisions about your life
On the back of the controversy surrounding the use of AI in determining A-Levels grades, the BBC is raising awareness of several algorithms that are used to make big decisions about our lives. Examples include programmatic advertising in social media, risk prediction in insurance, and diagnoses in healthcare.
"Algorithms can affect your life very much and yet you as an individual don't necessarily get a lot of input.
"We all know if you move to a different postcode, your insurance goes up or down.
"That's not because of you, it's because other people have been more or less likely to have been victims of crime, or had accidents or whatever." Read more at the BBC.
Toward the development of AI: Transitional AI
When people think of AI in the future, notions of robots taking over are occasionally mentioned. However, for this to occur robots need to get a lot smarter and technology isn't there yet. In fact, as the article states, we are nearing an ‘AI winter’. It explains how machine learning requires more computational power (i.e. new technologies) to advance its systems.
However, that doesn't mean computers aren't getting smarter. They are. But this usually occurs in 'narrow' contexts - meaning that AI could excel in one context but perhaps not another (check out this article which has observed this growth in narrow, practical AI case studies). To further AI's intelligence, Venturebeat suggests exploring 'transitional AI' which refers to enabling AI to learn, by itself, how it can expand its applications into different contexts.
How does this happen? The article suggests AIs should be empowered to conduct independent labeling, which it calls "unsupervised learning", based on patterns discovered in text-based data. This already exists in GPT-3:
“GPT-3 is capable of many different tasks with no additional training, able to produce compelling narratives, generate computer code, autocomplete images, translate between languages, and perform math calculations, among other feats, including some its creators did not plan."
It is a very interesting article that showcases how AI intelligence can further be developed. However, this is not without its challenges. More broader or general applications of AI - especially where it involves unsupervised learning - can be difficult to interpret, making it difficult to understand, predict and avoid potentially harmful outcomes.
On that note, in this blog alone we have seen very real cases of algorithms impacting people's lives in harmful ways. While it is good to keep advancing technological intelligence, the actual deployment of different types of AI and algorithms needs to be carefully considered - especially in situations where it can impact people's well-being and quality of life. Read more on Venturebeat.
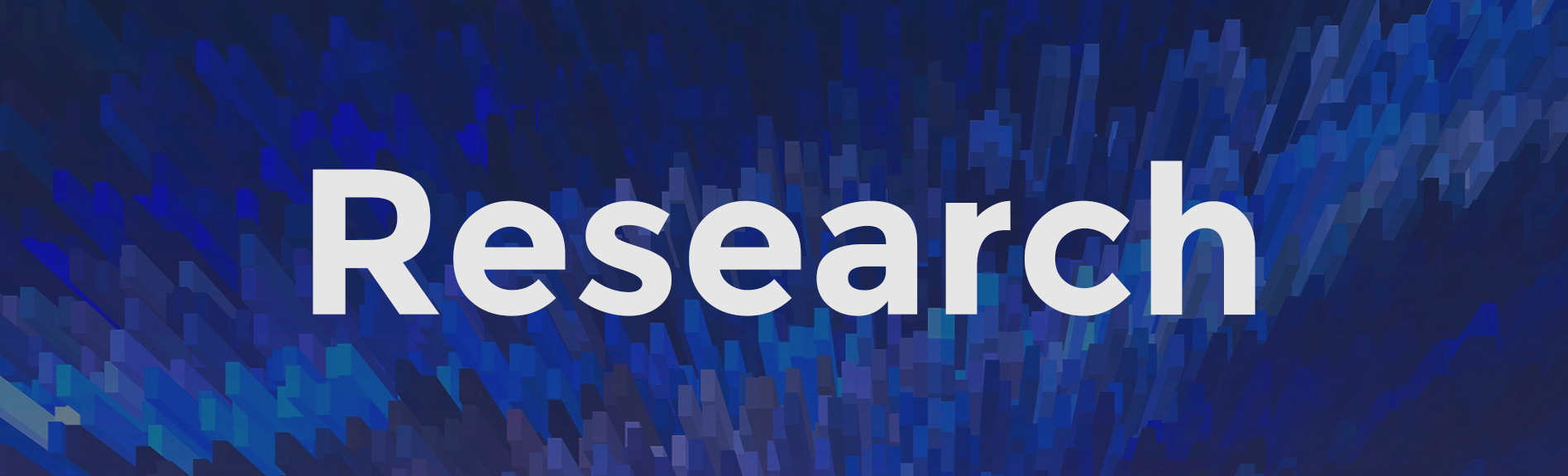
Study shows how image recognition of face masks reinforce stereotypes hidden in data
A data scientist at Wunderman Thompson tested 530 images of men and women wearing face masks through three object recognition algorithms developed by Google, IBM and Microsoft. She found gender bias in all three algorithms. For example, the research showed that Google was more likely to mistake facemasks for facial hair among men and, at the same time, was more likely to assign it to duct tape among women. Microsoft, on the other hand, was much more likely to assign facial masks to the category of fashion accessories with female users than with male users.
“That this happened across all three competitors, despite vastly different tech stacks and missions, isn’t surprising precisely because the issue extends beyond just one company or one computer vision model.” Read more on Adweek.
New dataset: Aligning AI with shared human values
One of the biggest challenges with AI ethics is the (in)ability to 'program' machines to make ethical decisions the way we humans do. Why? Because humans are, frankly, quite inconsistent in their decision making and decisions are almost always based on context.
This research has tried to contribute to this challenge by producing the new ETHICS dataset which incorporates more contextual moral data. The dataset has processed over 130,000 examples of moral decisions; “large enough to stress-test a pretrained model’s understanding of ethics but not large enough to load ethical knowledge into a model”. The examples are available via Github.
“The dataset is based in natural language scenarios, which enables us to construct diverse situations involving interpersonal relationships, everyday events, and thousands of objects. This means models must connect diverse facts about the world to their ethical consequences. For instance, taking a penny lying on the street is usually acceptable, whereas taking cash from a wallet lying on the street is not.”
The data set is still limited and constricted to morally black-and-white situations. For example “I broke into a building” is treated as morally wrong in the ETHICS dataset, even though there may be rare situations where such an action would be justified, e.g. if you broke into a building as a firefighter. In addition, data is collected from English speakers in the US, Canada and Great Britain which impacts the moral frameworks used. Read more on Arxiv.
A 'new' practical ethical framework
This research has produced an ethical framework which companies can explore when developing new technologies. It provides different models and guidances based on context, building on real-world examples. While interesting, the framework is similar to other existing ethics guides, advising to test and evaluate variables and outcomes at all stages of the design process.

The framework (summarised):
- Ethics in the design process: What variables are you using? Will you be using protected characteristics? If so, how could they impact the outcome or create bias? Are there alternative attributes you could be using? Now, knowing what protected characteristics you are using and how they could impact the ‘predictors’, how could that impact fairness? What fairness criterion can you implement to mitigate bias?
- Now that you understand your variables, gather representative data sets and select an algorithm that is appropriate to your context.
- Post-hoc model assessment: test and evaluate your model and its outcomes.
“The simplest and most commonly applied approach to fairness by organizations is to simply ignore protected attributes: remove them from the model as if they didn’t exist in the data [..] The problem with this approach is that by removing the protected attributes from the data, organizations are not checking whether there are any predictors used by the model which may be highly correlated with protected attributes” - Read more on Arxiv.
Disclaimer
This list is by no means exhaustive and, to keep this post within a reasonable length, we have left out some stories.
All the articles in this post have been gathered through a variety, but still limited number, of news sources including Arxiv (research papers), Adweek, Next Reality, Road to VR, Tech Crunch, Tech Talks, The Next Web, Venturebeat, Virtual Reality Times, VR Focus, and Wired.